Data Science and AI

Human-centered sustainable mobility
This activity aims at defining a new design framework for human-centered mobility systems, with active functionalities based on advanced control and optimization techniques that consider as input also social information shared by users, formally modeled thanks to opinion analysis tools. The study includes:
- Analysis of potential inclinations toward new mobility habits.
- Formulation of dynamic models describing the spread of new mobility habits.
- Integration of social dynamics into optimization as a feedback control, identifying optimal strategies for using low-cost incentives wtih the goal of maximizing user acceptance of energy and environmentally friendly mobility solutions.
E-Health & AI
We pursue original research in the area of digital health technology and AI. We develop and apply cutting-edge data analytics methods to extract actionable knowledge from health-related data, and we design novel digital systems to monitor, predict, and improve human health and wellbeing. For example, we develop AI algorithms to enable descriptive, predictive, and prescriptive health data analytics for several applications. We develop evaluation frameworks for mobile health apps in specific application areas, including automated methods to extract relevant apps’ features from large databases, and we contribute to the development of recommendations for app quality and for software as medical device (SaMD). We develop novel, data-driven online- and mobile-based approaches for hearing testing and rehabilitation. We develop algorithms, based on Natural Language Processing (NLP), Machine Learning, and medical ontologies to analyze large databases of clinical documents and health-related unstructured text. We develop integrated systems, using wearable and environmental IoT sensors and AI algorithms to monitor the individual health and wellbeing, and to enable individualized plans for treatment and follow up.
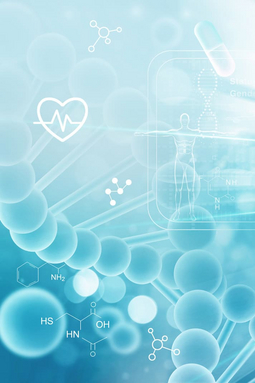

EXplainable AI
With the recent rapid advances in machine learning and artificial intelligence (AI), the attention of experts is increasingly turning to the impact of these fields on society. Awareness is growing of the potential risk of serious accidents, for example, design error, poorly specified goals or simple misapplication of the AI. The certification of an AI-driven system is therefore one of the open challenges of science and engineering for the near future. Some approaches to the problem deal with eXplainable AI (XAI). The objective of XAI is to ensure that an algorithm can explain its rationale behind certain decisions and explain the strengths or weaknesses of that decision. The research deals with the certification of AI, by focusing on: sensitivity analysis of XAI to drive scalability of formal logic and Lyapunov stability. Standards in automotive for risk analysis (SOTIF) and in avionics for design assurance, respectively, are studied in this perspective. Case studies in smart mobility and critical infrastructure are considered to build a data lake of realistic and challenging scenarios.
HPC for Big Data
Recent years have witnessed a deluge of data, deriving from even more complex scientific acquisition instruments and simulations, mobile sensing, Internet-of-Things, online social media, and many other sources, bringing people into the age of Big data. The management and analysis of these data require a vast amount of storage and computing resources, therefore High Performance Computing (HPC) is of great importance for extracting knowledge from them.
This activity focuses on the design of innovative solutions, based on state-of-the-art middlewares, frameworks and tools, enabling applications to take the most out of present, heterogeneous advanced computing platforms.
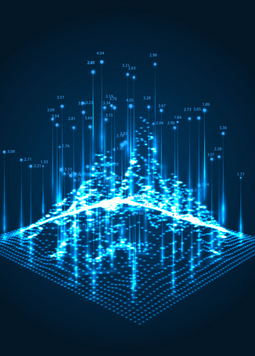